A Guide for Modern Data Strategies
As companies grow, their data needs often grow even faster. Traditionally, organisations have relied on centralised data teams and monolithic data warehouses to manage, store, and analyse data. However, with the rise of data mesh, many organisations are rethinking how they approach data architecture. Data mesh, an innovative approach introduced by Zhamak Dehghani in her groundbreaking book Data Mesh: Delivering Data-Driven Value at Scale, decentralises data management by pushing ownership and governance to domain-specific teams rather than a single, centralised group. While this approach has its advantages, it also introduces some challenges.
In this post, we’ll explore the pros and cons of data mesh to help you determine if it’s the right choice for your organisation.
So, what is Data Mesh in a Nutshell?
In a world where data drives decisions, the way we manage it can make or break business agility. Traditionally, organisations have relied on centralised data architectures where all data flows into a single warehouse or lake managed by a central team. While it may seem efficient initially, this setup often leads to significant friction. Domain teams, eager to analyse and interpret data, have questions that pile up, while the overwhelmed central data team struggles to address an ever-growing backlog. The result? Delays, bottlenecks, and frustration for everyone involved.
Here’s a visualisation of the traditional data architecture:
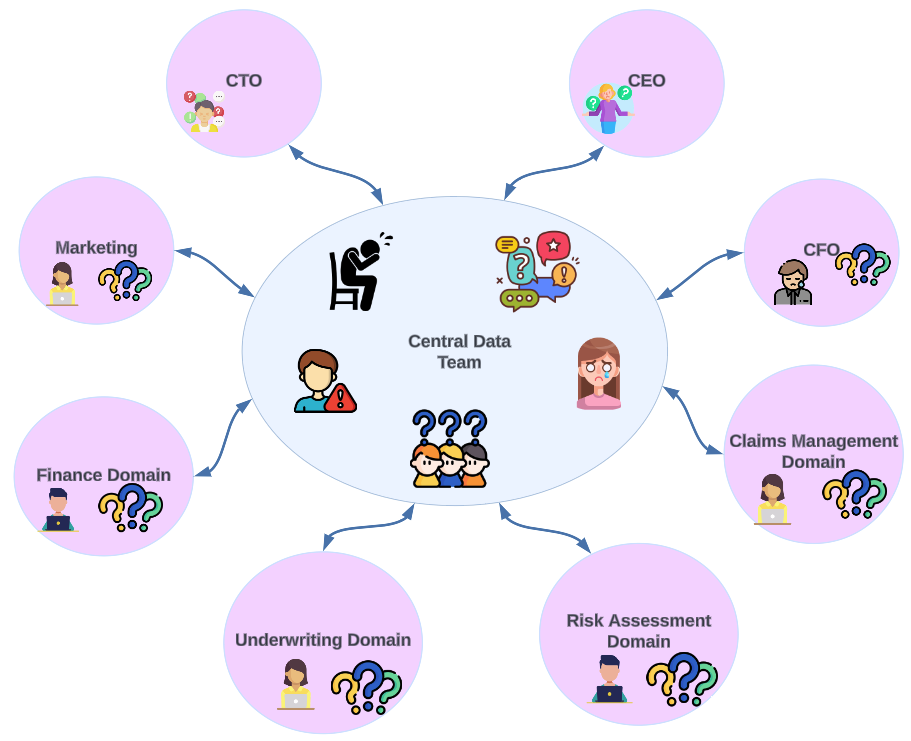
Now, take a look at the data mesh architecture. This decentralised model shifts the chaos into clarity by empowering each domain to manage its own data as a product. Domain teams, like Claims or Underwriting, become both producers and owners of their data, leveraging their expertise to ensure quality and relevance. A self-serve platform connects these domains, enabling seamless data sharing and discovery while adhering to governance standards. The result? Greater agility, scalability, and collaboration without the bottlenecks of a central team.
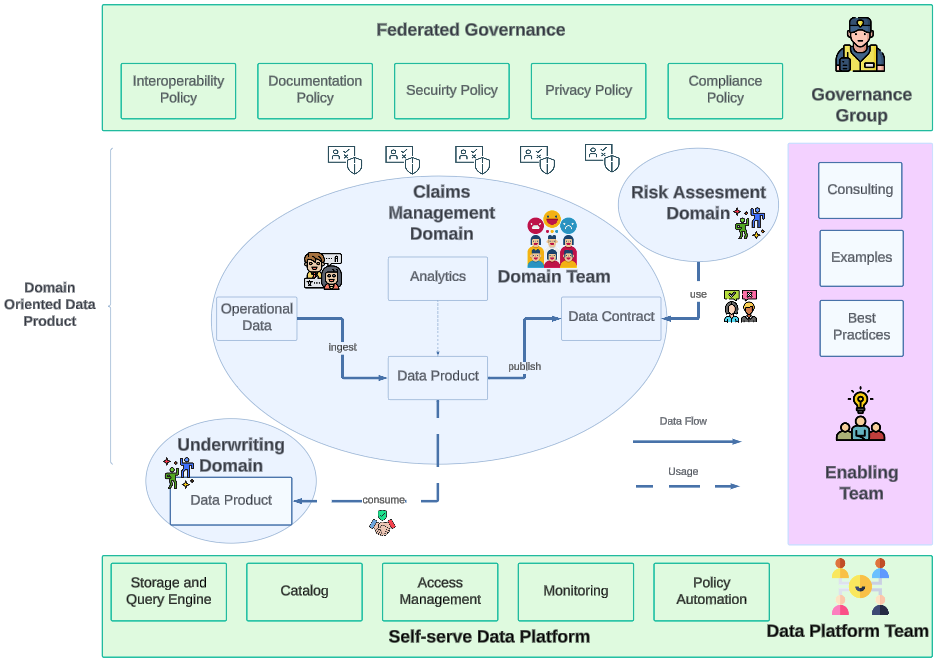
So, think of data mesh as a way of treating data like a product, where each domain takes charge of the data it generates and uses. Instead of centralising all data in a single warehouse or lake, data mesh allows domains to manage, transform, and share their data independently. However, it’s all done under a set of standard governance principles to keep things consistent across the organisation.
For example, in an insurance company, different domains like claims management, risk assessment, underwriting management, and finance play key roles. Claims management focuses on processing claims and tracking their history. Risk assessment analyses factors like natural disasters or market trends to guide underwriting decisions. Underwriting management handles data related to issuing, renewing, or cancelling policies, while the finance team manages financial data and profitability models.
Each of these domains is responsible for turning its data into reliable and accessible products that can be shared across the organisation. This decentralised approach encourages agility and scalability while maintaining governance and consistency.
Assuming we’re on the same page about what data mesh is, let’s dive into the good stuff, its pros and cons.
The Not So Great Things About Data Mesh
It’s Not Exactly Easy to Get Going
Switching to a data mesh isn’t as simple as flipping a switch. It means rethinking your entire data architecture, reshaping roles within teams, and changing how the organisation thinks about and handles data. This can be tough, especially if your organisation isn’t already strong on data governance. Getting data mesh up and running usually involves building new infrastructure, putting standard practices in place, and making sure teams are well-trained. It’s a big undertaking, and the time, effort, and costs can quickly pile up.
You’ll Need Stronger Governance
A decentralised data model means more people are involved in handling data, which can be great for speed, but it also makes governance a bit trickier. To keep things from turning into a messy web of disconnected data silos, you need clear guidelines on things like data security, compliance, and how different teams’ data will work together. Without these guardrails, there’s a risk that the data mesh could actually add to the chaos it’s meant to solve.
Data Duplication and Siloed Knowledge Are Real Risks
When each team takes charge of their own data, there’s a chance they’ll end up creating duplicate or even conflicting data products without realising it. If this goes unchecked, it can lead to inconsistencies that mess with the accuracy of insights across the organisation. On top of that, each team could end up with its own way of interpreting and documenting data, making it harder for other teams to use or understand their data. This can limit collaboration and slow things down.
More Work for Domain Teams
With data mesh, domain teams take on responsibilities that would usually fall to a centralised data team, like data processing, storage, and even compliance. While this makes sense in theory, it can be overwhelming, especially if those teams aren’t well-equipped with the right skills or resources. To pull this off, you might need to bring in new team members or invest in training, which takes time and money. It’s a lot to ask from teams that are already juggling a lot of other responsibilities.
Tooling Can Be a Bit Behind
Since data mesh is a relatively new approach, not all the tools out there are built to support it yet. Many existing data tools are designed for centralised systems, so they don’t always mesh well with the decentralised approach of a data mesh. This could mean having to customise existing tools or even build new ones from scratch, which adds another layer of complexity and cost.
What’s Great About Data Mesh?
Scaling Without the Bottlenecks
Picture a centralised data team trying to manage and prioritise requests from every department. As demand for data grows, so does the backlog. The teams generating data are overwhelmed with endless requests, while data consumers are stuck waiting, often frustrated by delays. The more they want insights fast, the more the central team becomes a bottleneck, creating a cycle where no one feels fully satisfied.
In a traditional setup, this can lead to some real friction. Domain and operational teams are all doing their own thing, while the central data team is swamped, and consumers of data are just waiting around. Everyone wants to prioritise their own needs, but the central team’s backlog slows things down for everyone.
Data mesh fixes this by enabling each domain team to manage its own data while leveraging a self-serve data platform. This platform allows both data producers and consumers to share and access data without constantly relying on a single overstretched team. By decentralising ownership but keeping everything connected through the platform, organisations can scale efficiently and empower teams to work independently without feeling like they’re offloading work. The platform ensures consistency and connectivity across teams, providing a foundation of standards and governance that prevents silos and maintains harmony as the organisation grows.
Tapping Into Domain Expertise for Better Data
Centralised data teams know a lot about managing data but might not fully understand the specific details of every area they support. That gap can lead to misunderstandings or even mistakes that impact decisions.
Data mesh changes things by putting domain experts, whether they’re in claims management, risk assessment, policy management, or finance, at the helm of their own data. These teams already know their data inside and out, so they can apply the best checks, transformations, and standards to ensure high quality. This means data is more reliable, more accurate, and more likely to lead to solid, informed decisions. In a data mesh setup, each domain has data owners who are in charge of their data products and making sure they meet certain quality standards, like SLAs (service-level agreements). These data owners also make it easier for data consumers to get answers or insights, compared to relying on a big, centralised data team. It’s all about making things smoother and more collaborative.
Built for Flexibility and the Future
Tech moves fast, especially in data. Many traditional data systems aren’t built to keep up with new tools, frameworks, and platforms. Data mesh, however, is designed to be adaptable. It’s easy to plug into cloud-based tools, big data frameworks, and machine learning platforms as they evolve.
With data mesh, teams don’t have to wait on a central data group to update a giant system. Each domain team leverages a shared data platform to ensure consistency and interoperability. This balance allows teams to innovate while staying grounded in a cohesive organisational strategy.
Real Ownership and Accountability
In many traditional setups, it’s hard to say who’s really in charge of what. When ownership and accountability are unclear, data quality and governance tend to slip through the cracks.
Data mesh fixes this by giving each domain team clear ownership of its data. When a team knows it’s responsible, they’re far more likely to stay on top of quality, security, and compliance. This setup encourages teams to take good care of their data since they’re directly accountable for what they produce and share across the organisation.
So, Is Data Mesh Right for Your Organisation?
Now that we’ve covered the pros and cons of data mesh, the next big question is: Is it the right solution for your organisation?
Data mesh brings a fresh approach with some impressive benefits, but it also comes with its own set of challenges. It’s definitely not a one-size-fits-all solution. Whether or not it’s the right fit for you really depends on your organisation’s specific needs, resources, goals, and mindset. A great way to evaluate its potential is by starting small consider running a Proof of Concept (PoC) or an experiment within a single domain or team. This allows you to test the approach, gather evidence, and measure outcomes such as reduced bottlenecks, improved data sharing, and enhanced insights. The lessons learned can guide you in scaling the model or adjusting your strategy.
Think of adopting data mesh as more of a journey than a one-time decision. It’s a way to tackle some familiar obstacles, plan for the new challenges that will come, and ultimately move towards better, more scalable data management. Many companies are already embracing this journey, seeing it as a path to a more agile and forward-thinking data culture.
For this journey to work, a certain mindset is key. If your organisation values flexibility, innovation, and growth, then data mesh could be a great fit. With the right attitude, even the challenges that pop up along the way can be turned into opportunities. But, if your company culture tends to resist change, this shift might be tougher to pull off. In those cases, it might make sense to hold off on diving into data mesh until the environment becomes more open to change.
Wrapping It Up
At the end of the day, data mesh represents a big shift in how organisations think about and manage their data. Decentralising data ownership and tapping into domain expertise offers some pretty exciting benefits like better scalability, quicker insights, and more reliable data quality. But, like any big change, it also comes with its own set of challenges, especially when it comes to implementation and ensuring strong governance.
Taking the time to understand both the pros and cons will help you make a more informed decision about whether this approach aligns with your data-driven future. And keep in mind this is a long journey, one that’s easier to navigate with the right expertise and support.
At fourTheorem, we’re here to support you every step of the way. Our Data Mesh Accelerator allows you to rapidly pilot and explore the benefits of a data mesh in a risk-free environment, leveraging proven templates and deep expertise in modern data services. This solution is tailored for organisations facing challenges in data management and scalability, embarking on significant migration and modernisation efforts, or seeking to unlock innovation and leverage GenAI use cases.
By partnering with us, you’ll have the tools and expertise to navigate this transformative journey with confidence and success. Contact us today to get started.