GenAI RAG
Harness the power of Generative AI Retrieval Augmented Generation (RAG) for your business.
What is RAG and Q for Business?
Retrieval Augmented Generation (RAG) is a natural language processing technique that enables generative AI models to reference an authoritative knowledge base before generating an answer. RAG can be used to build AI assistants tailored to a specific business domain. Amazon Q for Business enables the rapid creation, tuning and deployment of RAG solutions.
Self-build RAG requires deep expertise in LLMs, significant investment in hardware and software, ongoing maintenance, robust security and data management to ensure system accuracy.
Benefits
Team Productivity
Q for Business streamlines workflow by summarising documents, generating drafts, conducting research, or running comparative analysis.
Tailored to Your Needs
Q connects to company information, allowing it to generate content and take actions that are relevant to your business.
Secure & Compliant
Q understands and respects existing role and permission structures and boundaries, enabling customers to meet their most stringent enterprise compliance needs.
Reduced Code & Infrastructure
Compared to self-build, a Q implementation has a far lower implementation and maintenance overhead, reducing TCO.
Constantly Evolving
The LLM technology backing Q is constantly evolving, meaning that improvements can be incrementally deployed at low cost.
Sustainability
Using centrally trained and managed models, as opposed to self-training Q improves your company’s carbon footprint.
GenAI RAG Accelerator
Rapidly pilot and explore the benefits of Generative AI for your business by leveraging off-the-shelf templates and our expertise in building custom AI solutions.
Tailored for organisations with internal domain-specific knowledge bases; for example, standard operating procedures and market intelligence.
How it works
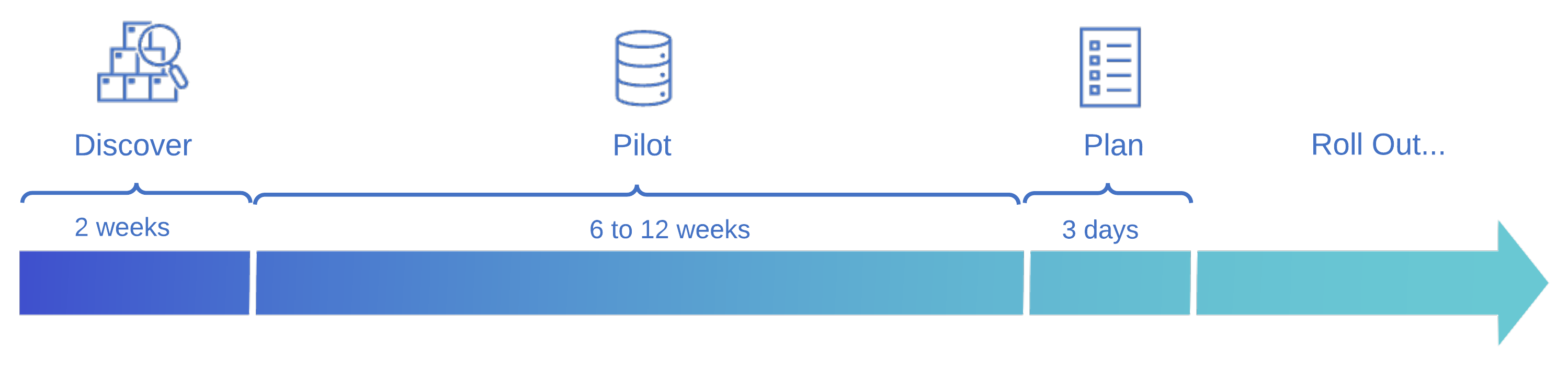
1. Discovery
The RAG accelerator beings with an initial discovery workshop which typically takes one day. The aim of the workshop is to identify high business value use cases. Following the workshop, we determine the which of the identified use cases to pilot based on the availability of data, security and compliance requirements and end user needs. We also determine a set of objective business KPIs to measure during the pilot. In total this phase takes takes approximately one week.
2. Pilot
During the pilot phase, we configure and deploy the Q RAG application(s) along with the associated infrastructure required to securely connect to data sources and end users and to measure the identified KPIs. Deployment of the pilot typically takes two to three weeks. We then allow time for users to engage with the assistant to collect feedback.
3. Plan
Objective KPIs are measured and, along with subjective user feedback we evaluate the business impact of the pilot. Based on these measurements we determine a rollout plan to move to a full production implementation. The plan typically addresses issues such as data security, classification, versioning and role-based access.
4. Roll Out
Post pilot, we can support secure and compliant rollout of the RAG solution across the organisation.
Why fourTheorem? We wrote the book on it.
AI as a Service is a detailed guide to operationalising Cloud Native AI Services on AWS.
As an Advanced Consulting Partner, we have architected and delivered systems for our customers that harness the power of AWS AI services.
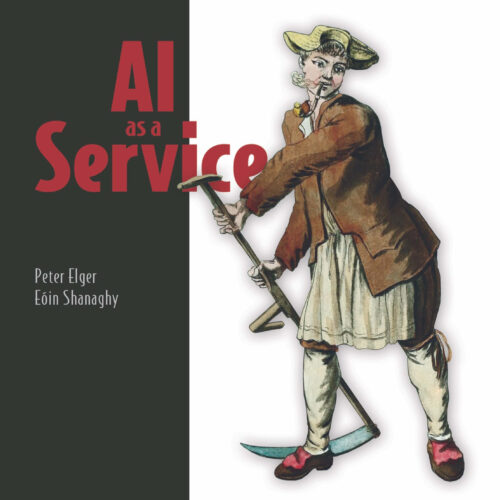
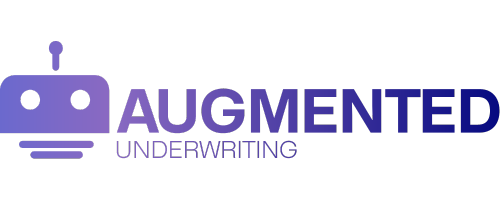
The AI-Powered Underwriting Assistant.
Our Augmented Underwriting RAG assistant has been trained on 24 years of Lloyds of London public market bulletins.
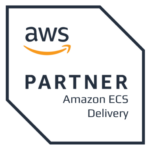
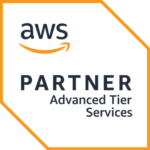
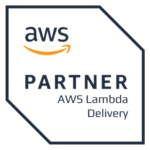